1.
Overview
2.
Basic Ideas: Information and structure, Attractors and Repellors
3.
Basic Ideas: Catastrophe
4.
Structure and Chaos
5.
Six Kinds of Replication
6.
Categories and Logic
7.
Surprise and importing structure
8.
Replication
|
Catastrophe
The idea of catastrophe is another fundamental notion once fashionable
in cognitive theory (for example, the whole issue of Behavioral Science,
1978, vol 23 No. 5 was devoted entirely to "Applications of catastrophe
theory in the behavioral and life sciences").
To define a catastrophe there must be variables of two classes:
call them "input" and "output," or "state" and "consequence." In
a non-catastrophic relationship, each possible value of the input
variables corresponds to a unique value for each output variable.
In a castrophic relationship, the output has more than one value
for a given input over at least part of the input range. Moreover,
there are limiting values of the input at which the output changes
from multi-valued to single-valued, and at which at least two of
the multiple values are distinct. If the input moves across a limiting
value while the output is at a value different from the output on
the single-valued part of the function, the output moves abruptly
(catastrophically) to the new value
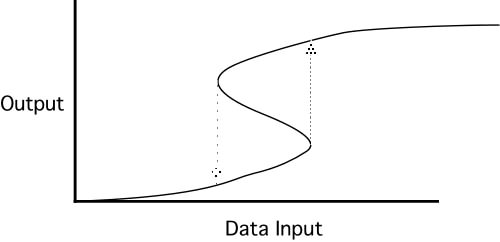 |
Fig 2. A function that shows a fold catastrophe.
As the input data move up from zero, the output stays on
the lower part of the curve until it abruptly switches to
the upper curve. But when the data input goes down again,
it does not return to the lower part of the curve until a
lower value is reached than the one at which the output flipped
on the way up. The function shows "hysteresis".
If in some way the input and output are made to take values
on the intermediate "reverse" part of the curve,
the slightest disturbance will cause the output to flip to
the outer branch in the direction of the disturbance. The
outer branches are stable "attractors", the inner
on a metastable "repellor". |
Figures 1 and 2 represent one-dimensional input and output variables
with, respectively, no catastrophe and a "fold" catastrophe. If a
second input variable is added that controls a transition between
these two states, it may generate a function known as a "cusp" catastrophe.
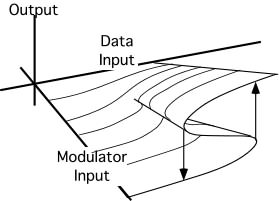 |
Fig 3. A cusp catastrophe generated
by a modulator, or "stress" variable. At low stress,
the output varies smoothly with the data input, but as the
stress increases, the output must take on values from one
or other of the outer branches of the embedded fold. |
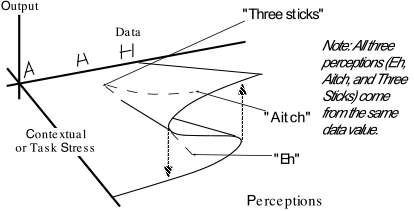 |
Fig 4. Example of cusp in the perception
of three (possibly related) lines. With little stress, the
perception is of three lines angled with respect to one another.
When there is stress to produce a "perceptual decision" the
perception is of either "A" or "H". |
Cusp catastrophes will be shown to be important in learning, and
to provide a route between the chaos of alertness on the one hand,
and the stability of categoric perception or the precision of logical
thought on the other.
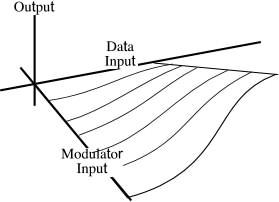 |
Fig 5a. At an early stage of learning, there is no discrimination, although
the learner can perceive differences along the data dimension, and the
output may be stronger as the modulator input (stress) increases. |
Fig 5b. As learning begins, the learner is able to make choices between
the two categories of item, but does so only when the modulator input
is strong, and a decision is forced by either context or task pressure. |
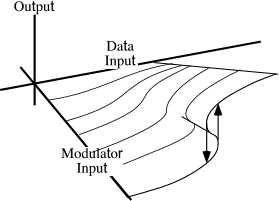 |
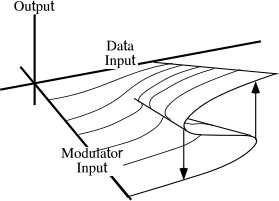 |
Fig 5c. When the discrimination is well learned, the learner will tend
to make the discrimination even with very little pressure to do so. The
data will "automatically" be seen as representing one of the
learned categories, rather than just as a variable data value. |
The modulator input might be pressure from a teacher to make a decision as
to which category is represented by the data,it might be local context (e.g.
the "three-stick" figure is likely to represent a letter A or H
if it is surrounded by other letters such as C*T or T*E), or it might be
a "need to act" in some situation. Decisions are easier and occur
with less modulator stress if the discrimination is well learned.
|